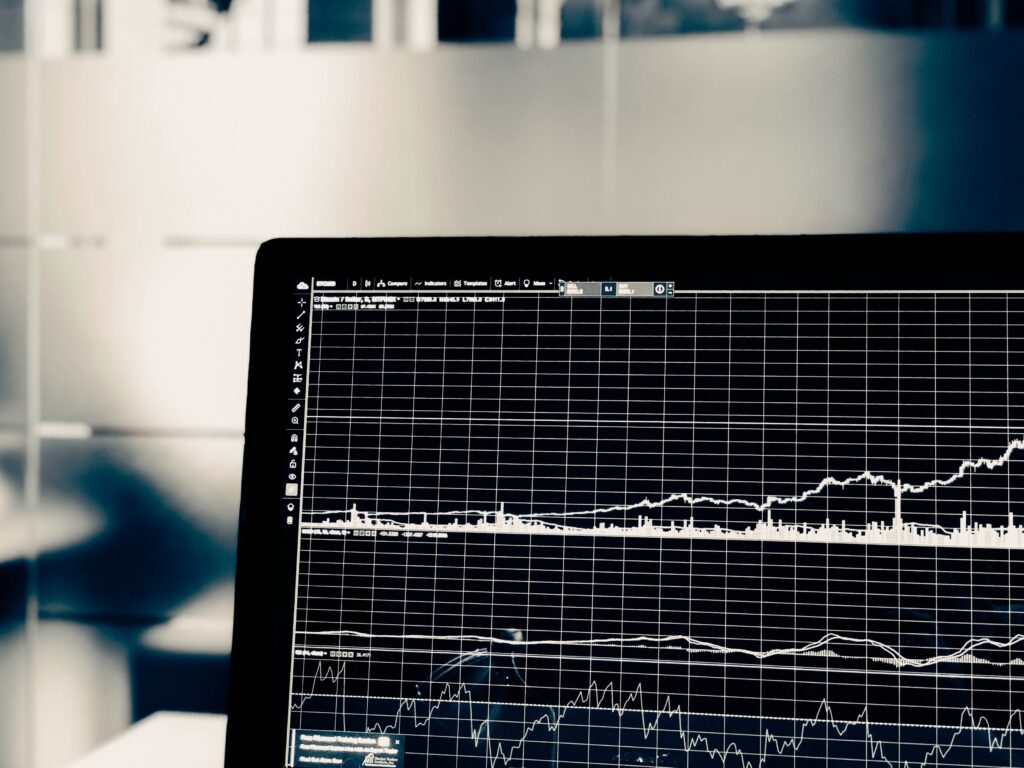
As people develop more advanced technologies to help their lives, artificial intelligence (AI) comes into the fray as one of the trademark technologies of the modern era. In particular, machine learning (ML) has become one of the forefront examples of AI technologies currently in use in many industries, including healthcare. However, machine learning technology is not foolproof, considering the risks of inequity and biases in the healthcare industry.
In May 2023, researchers from the University of Maryland School of Medicine (UMSOM) discovered that the majority of crowdsourced datasets in prestigious medical imaging data science competitions to develop AI did not include demographic data. In addition, these datasets were not assessed later on to check for potential instances of inherent biases.
About 61% of datasets from 23 data science competitions do not include demographic-related information such as age, race, and sex. If the data used in machine learning are biased (i.e. The data is flawed in some way which can cause systemic errors in machine learning outputs), processes that rely on machine learning outputs can become biased as well. Within a healthcare context, population demographics with a history of underdiagnosis and/or under-treatment will likely also be underrepresented in machine learning training datasets. Consequently, healthcare machine-learning models may perpetuate this inequity.
How Can Machine Learning Amplify Biases and Inequities
If designed properly, machine learning models are capable of enhancing the disease detection and diagnosis abilities of human clinicians. For example, machine learning models can accurately predict the amount of remaining years a person has before their death or detect Alzheimer’s disease from an early age.
However, machine learning techniques are not infallible. Since machine learning technology is still in its nascent phase, the models are often trained on data commonly collected from people with easier access to healthcare. This practice can lead to the development of biased ML models. In determining the potential exacerbation of biases in machine learning models, a group of MIT scientists brought forth four variables, namely attribute imbalance, class imbalance, attribute generalization, and spurious correlations.
An example of bias in the machine learning models’ procession of images is the conception of class or attribute. Picking cows and camels as two examples of classes, the scientists displayed how attributes of these animals are not specifically tied into both classes.
By taking grass and sand as examples of attributes, the machine learning models could reach erroneous decisions by concluding that cows do not live in sandy places. The contrary is also the case, in which the models may conclude that camels do not live in grassy plains.
The possibility of making erroneous conclusions will be disastrous in determining the possible causes of illnesses and the proper solutions to address them. Attribute generalization would also be dangerous in that it is capable of creating the incorrect generalization that certain groups of people are more susceptible to illnesses whereas others would not be afflicted. This can result in the underdiagnosis and treatment of individuals from certain populations.
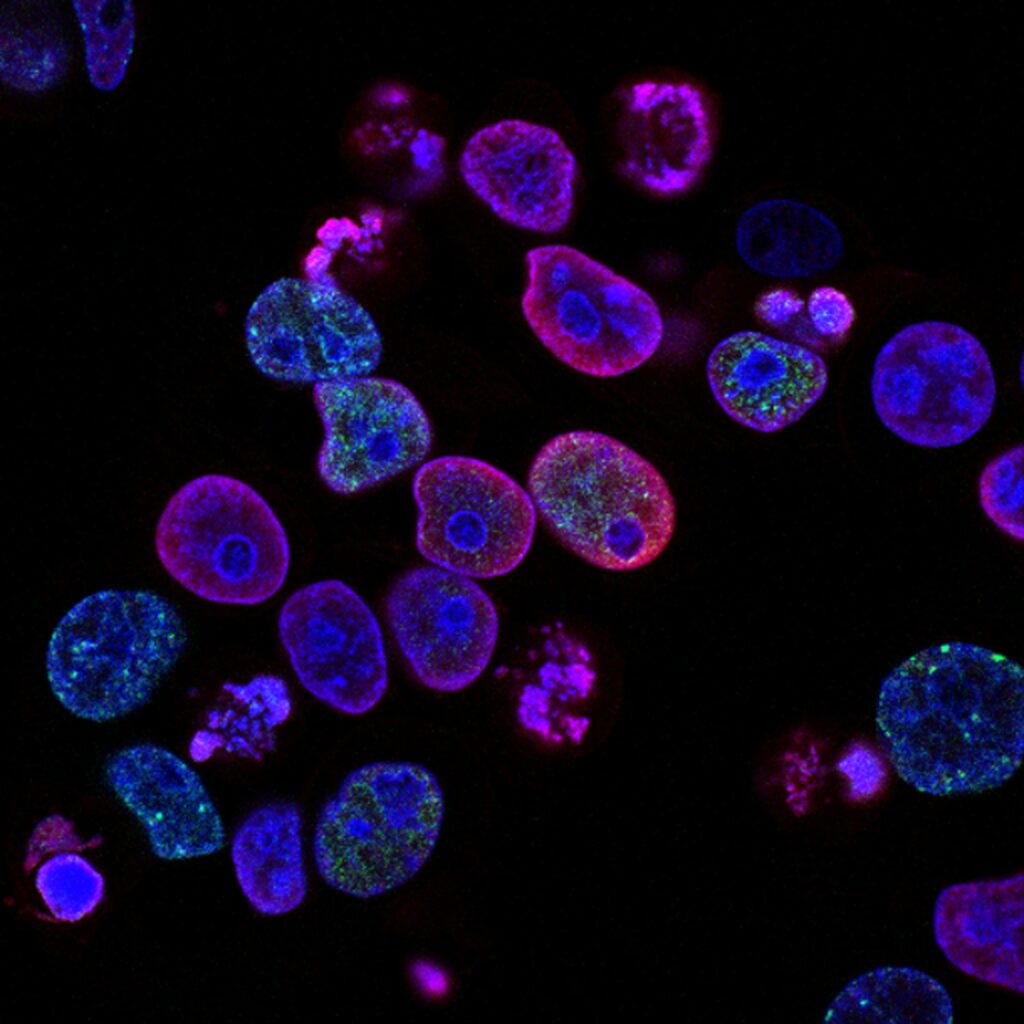
Errors and Issues in Datasets for Machine Learning
In discussing the potential drawbacks of using machine learning models in healthcare, several errors and issues have been identified. As previously mentioned, many of the data sets used in the US healthcare industry to develop machine learning models did not involve demographic data such as age, race, and sex. This weak spot may unintentionally discriminate against underrepresented groups who also deserve to access reliable healthcare services.
The issue of feedback loops is also evident in which the machine learning models will suggest the same kind of healthcare content to a specific person based on their past behavior. Over time, this behavior can change, and yet the system may decide to provide the same kind of content regardless of the person’s behavioral changes.
Algorithmic fairness is also one of the most challenging issues in machine learning models that experts have to deal with. The reason is that everyone has their own conception and understanding of fairness, and what is fair to one specific group of people will be considered unfair to others.
If the models only relied on the existing and flawed data sets, predictive accuracy would hamper the healthcare industry’s ability to ensure equal opportunity for everyone in society.
Lastly, the issue of data drift will become harmful in the long run if experts do not update the datasets that the machine learning models rely on. To reduce prediction inaccuracies, these models have to be regularly updated and retrained. If not, the machine learning models will continue to rely on outdated and biased data and will not be able to address the current healthcare concerns accurately.
Implication for Healthcare Development in Southeast Asia
As machine learning models are the recent additions of digital technology in the healthcare industry, AI/ML experts and healthcare professionals must work hard to address the potential weaknesses of these models to combat biases and inequity.
Addressing these issues will not be the only responsibility of the healthcare industry in modern countries, but also every single country that will eventually use AI and machine learning models to boost healthcare services.
Despite the problems poised in healthcare services in Southeast Asia, the industry in the region slowly embracing the use of machine learning and other AI technologies to improve livelihoods, including healthcare. Since using AI to boost healthcare services has become an inevitability, healthcare professionals in the region will need to address the issues of biases and inequity specific to the region for the sake of an inclusive and all-encompassing healthcare industry.
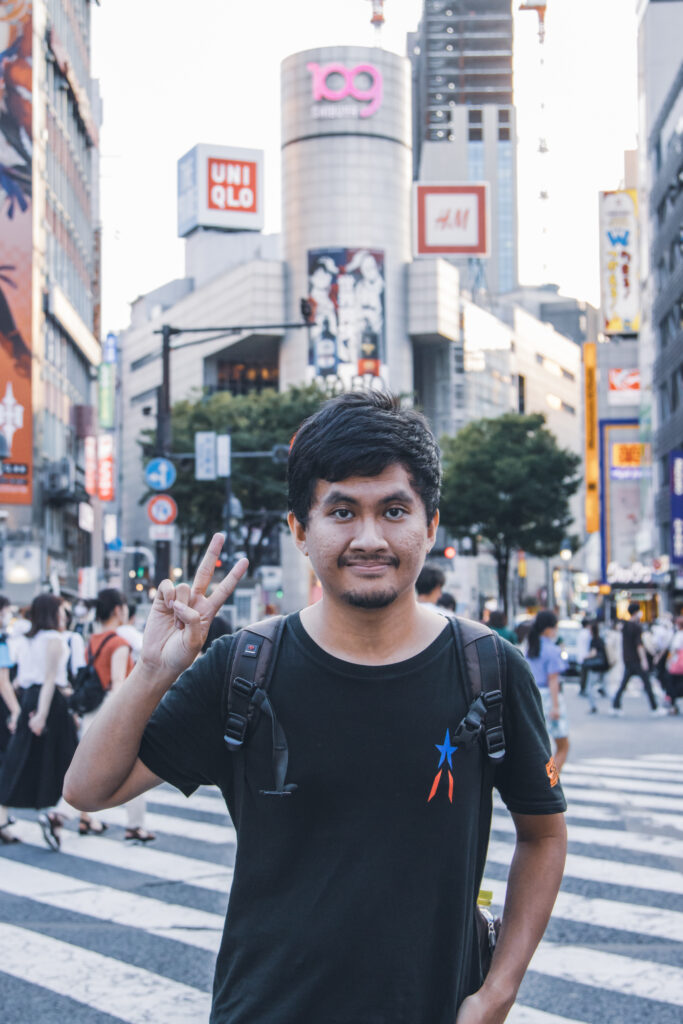
About the Author
Rizqi Wisnu Mauludino
Goes by Rizqi, he is a passionate writer from Indonesia with an interest in just anything that catches his attention. Captivated in so many topics ranging from foreign policy to IT as well as anything related to pop culture. Looks forward to being a specialist in writing articles about Japanese studies.